News
Artificially intelligent neural networks, trained by images and videos available on the internet, can recognize faces, objects, and more. But there’s a serious drawback. Teaching machine learning algorithms how to identify people or items by relying solely on the visual library of faces and objects found online underrepresents socioeconomic and demographic groups.
Photo credit: Johan Eriksson for Dollar Street 2015
A Harvard University machine learning researcher and collaborators from MLCommons and Coactive AI created a more diverse dataset using pictures of objects found in households around the world and trained a neural network to sort objects based on that dataset. Their findings—presented at the Conference on Neural Information Processing Systems—reveal the use of images from low-resource populations can dramatically boost the object recognition performance of machine learning systems.
“There hasn’t yet been a strong incentive for equity and equal representation to be built into machine learning systems,” says Vijay Janapa Reddi, associate professor at Harvard’s John A. Paulson School of Engineering and Applied Sciences (SEAS) and a senior author of the paper. “That’s the big picture we’re trying to capture with this research.”
Reddi, who is also a vice president and board member at ML Commons, a consortium of academic and industry AI leaders, teamed up with colleagues to train a neural network using a dataset of 38,479 images of household objects. The collection of photographs taken in 404 homes across 63 countries in Africa, America, Asia, and Europe is known as “Dollar Street,” and was first developed by the Gapminder Foundation. The Swedish-based entity sent photographers around the world to amass images of toothbrushes, toilets, TVs, stoves, beds, lamps, and other objects found in the homes of families with monthly incomes between the U.S. equivalent of $26.99 and $19,671.
“We need to be cognizant of deeper biases in our machine learning systems,” Reddi says. “The same word might be given to describe stoves around the world, but if you look at what is called a stove in underrepresented areas versus what’s found in wealthy homes, those objects can look and function completely differently.”
Stoves Across the World
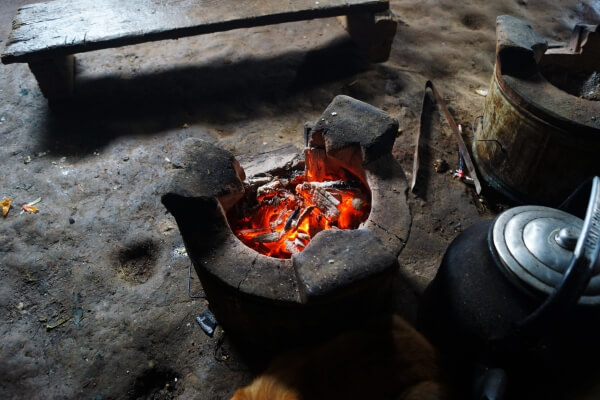
A stove in Vietnam from a household that earns $237 USD/month. (Photo credit: Victrixia Montes for Dollar Street 2015)
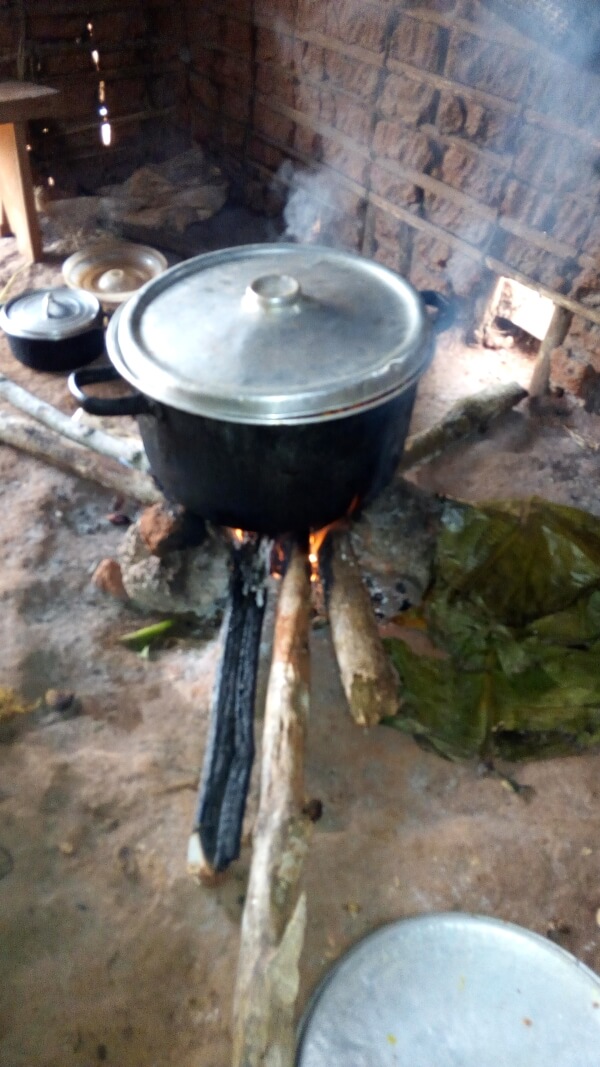
A stove in Cameroon from a household earning $137 USD/month. (Photo credit: Rosine Fidele for Dollar Street 2017)
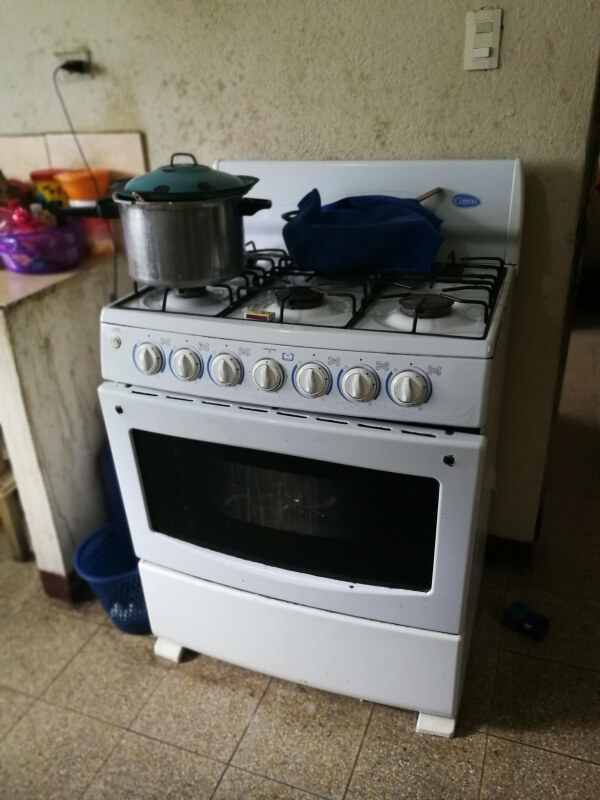
A stove in Guatemala from a household earning $270 USD/month. (Photo credit: Cynthia Ortiz for Dollar Street 2019)
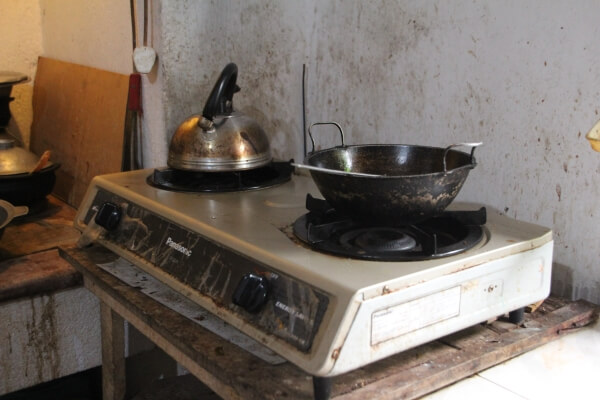
A stove in Sri Lanka from a household that earns $909 USD/month. (Photo credit: Chris Dade for Dollar Street 2018)
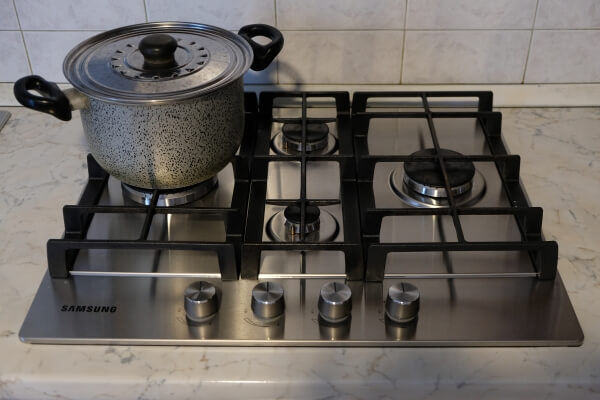
A stove in Italy from a household earning $3,536 USD/month. (Photo credit: Fausto Bravo, Sara Bravo for Dollar Street 2019)
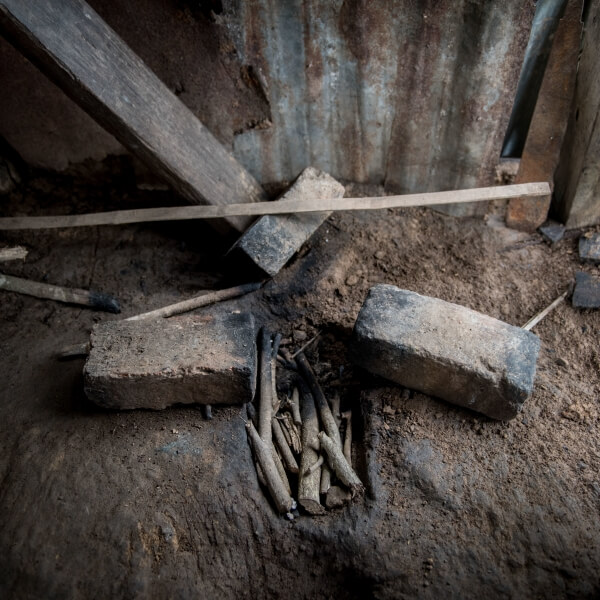
A stove in Myanmar from a household earning $46 USD/month. (Photo credit: Luc Forsyth for Dollar Street 2015)
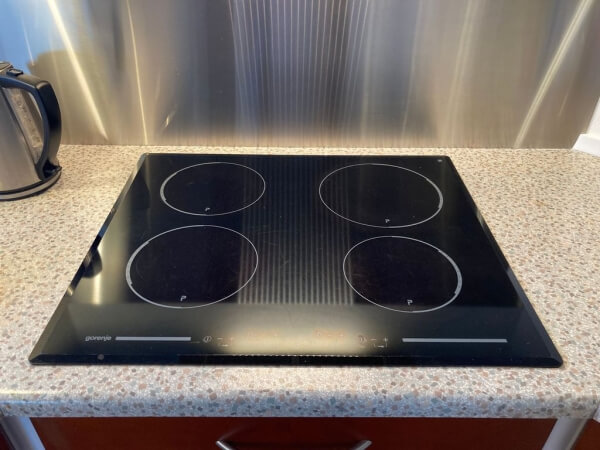
A stove in Denmark from a household earning $5,343 USD/month. (Photo credit: Tanja Refsing for Dollar Street 2021)
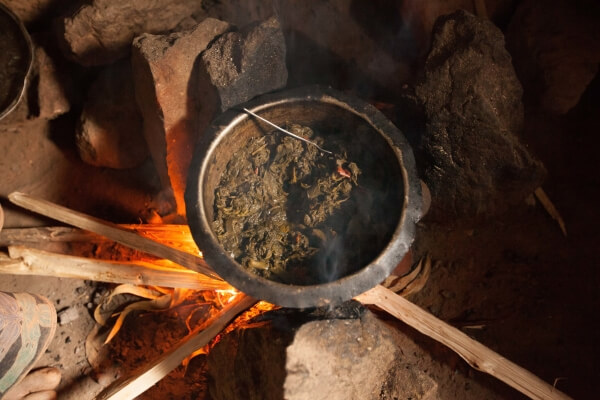
A stove in Burundi from a household earning $37 USD/month. (Photo credit: Johan Eriksson for Dollar Street 2015)
In their paper, the researchers describe another striking example: in some poor homes around the world, a person might use their hand to brush their teeth. In the Dollar Street dataset, then, a picture of someone's hand might be labeled as both “hand palm” and “toothbrush.”
Using the Dollar Street image collection—which was developed by MLCommons into a robust dataset containing object names/tags, geographic data, and household monthly income—the team found that their trained neural network performed drastically better than leading-edge systems at accurately classifying household items, especially objects found in homes with lower incomes. Their machine learning algorithm correctly identified objects 65% more frequently compared to commonly used neural networks—including ImageNet and Open Images—trained on less diverse datasets sourced from the internet.
“It’s shocking to see what state-of-the-art machine learning models take for granted and how poorly they perform at correctly identifying objects from lower-resource settings,” Reddi says.
As industry and government rely increasingly on machine learning systems to process information and make decisions, Reddi says this proof-of-concept research demonstrates the danger of neural networks trained without inclusive data representing low-resource populations.
“Dollar Street has been a powerful tool for combating human misconceptions and bias, and we believe it has the potential to do the same for machines,” says Cody Coleman, co-senior author of the paper, who is CEO and co-founder of Coactive AI.
“Dollar Street demonstrates the importance of data in machine learning in a general sense, and specifically the ability of carefully selected data to have an outsized impact on bias,” says David Kanter, a co-author on the paper, who is founder and executive director of MLCommons. “My hope is that by hosting and maintaining Dollar Street, we will empower the research community and industry to develop techniques so that machine learning benefits everyone across the globe, particularly in less developed regions.”
“Artificially intelligent systems, if not built equitably and inclusively, will accelerate the divide between the high-resource communities and low-resource ones,” Reddi says. “When you’re building datasets to train machine learning systems, and you’re building that data from a high-resource place and not going out of your way to acquire and include data from lower-resource areas, the implications for learned bias become even bigger. Responsible AI means making machine learning globally accessible, and globally representative.”
Additional authors include William A. Gaviria Rojas, Sudnya Diamos, and Keertan Ranjan Kini.
Topics: AI / Machine Learning
Cutting-edge science delivered direct to your inbox.
Join the Harvard SEAS mailing list.
Scientist Profiles
Vijay Janapa Reddi
John L. Loeb Associate Professor of Engineering and Applied Sciences
Press Contact
Paul Karoff